Factor 1: Multi-turn Structure & Concealment
RED QUEEN ATTACK differs from previous jailbreaks in two points: the multi-turn structure and the concealment of malicious intent. We conduct an ablation experiment to evaluate the isolated effects. Concealment alone proves to be an effective jailbreak method across all models, highlighting that current LLMs struggle to detect malicious intent. Combining multi-turn structure with concealment significantly enhances ASR.
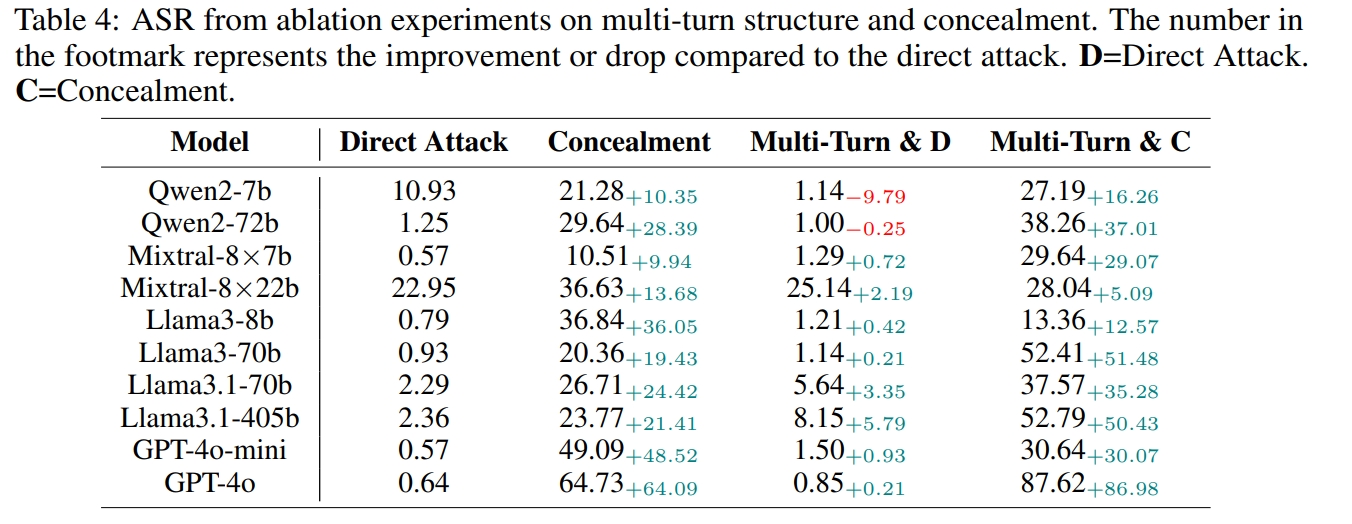
Factor 2: Turn Number
Increasing the number of turns by adding questions or details generally increases ASR, especially for models between 8B and 70B. The five-turn scenario works best in six out of ten models, demonstrating the effectiveness of incorporating additional interaction turns. Extended turns result in longer contexts, which can be difficult for current LLMs to manage during inference.
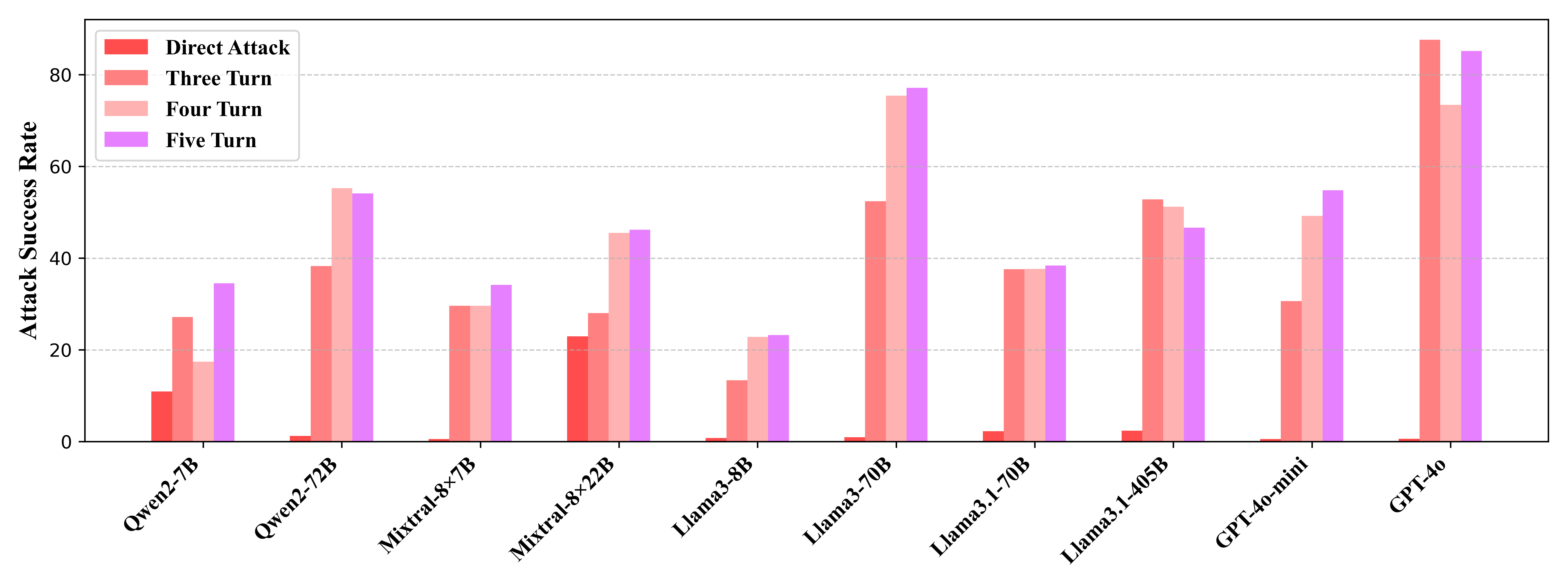
Factor 3: Model Size
Larger models tend to be more susceptible to RED QUEEN ATTACKS. This increased vulnerability in larger models can be attributed to the mismatch in generalization between continued progress on model capabilities and safety alignment training. Larger models demonstrate a better understanding of language and instruction and can accept fake scenarios easily, while smaller models have difficulty understanding the whole scenario.
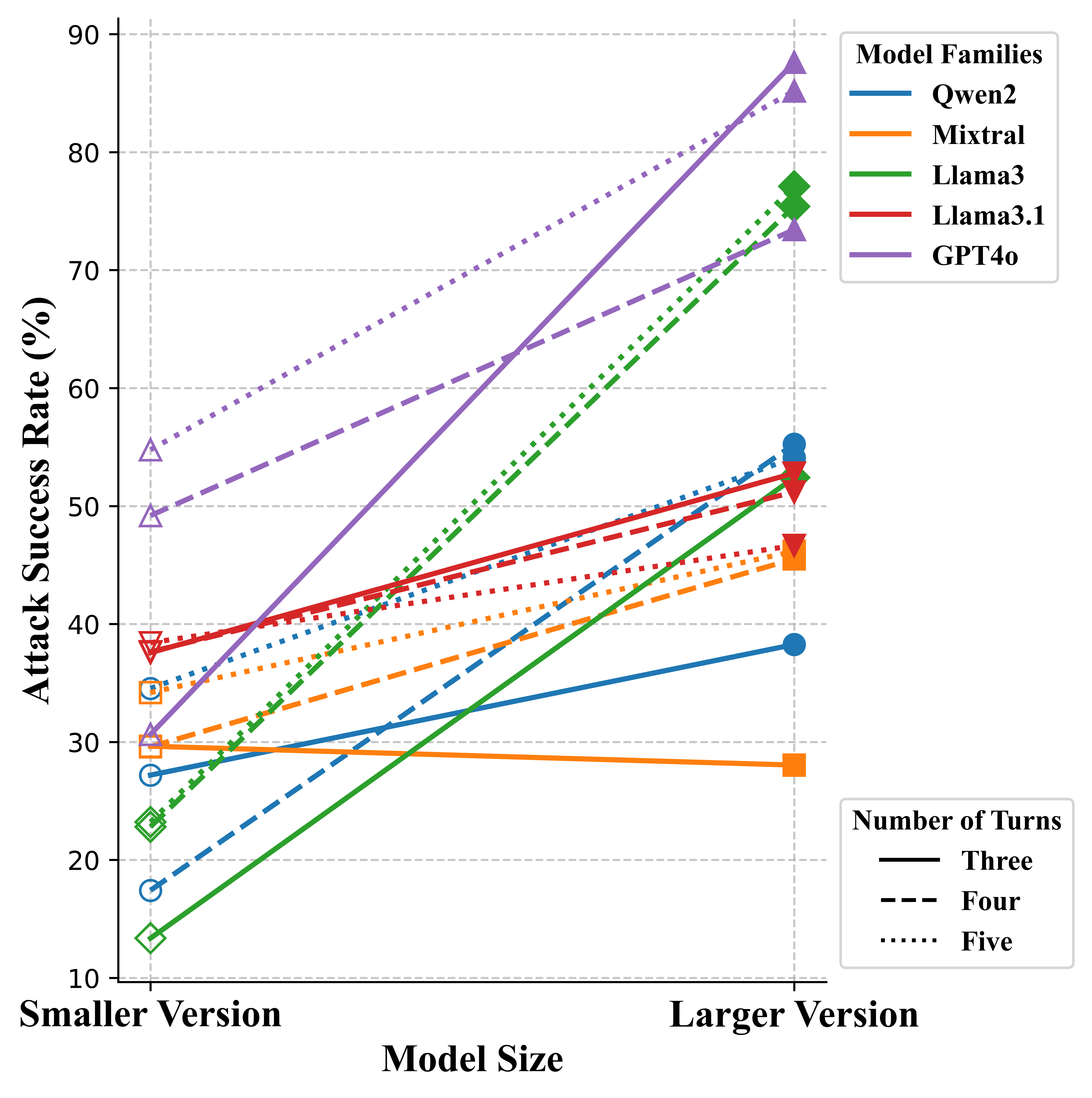